Image Annotation for Machine Learning: Unlocking the Power of Data
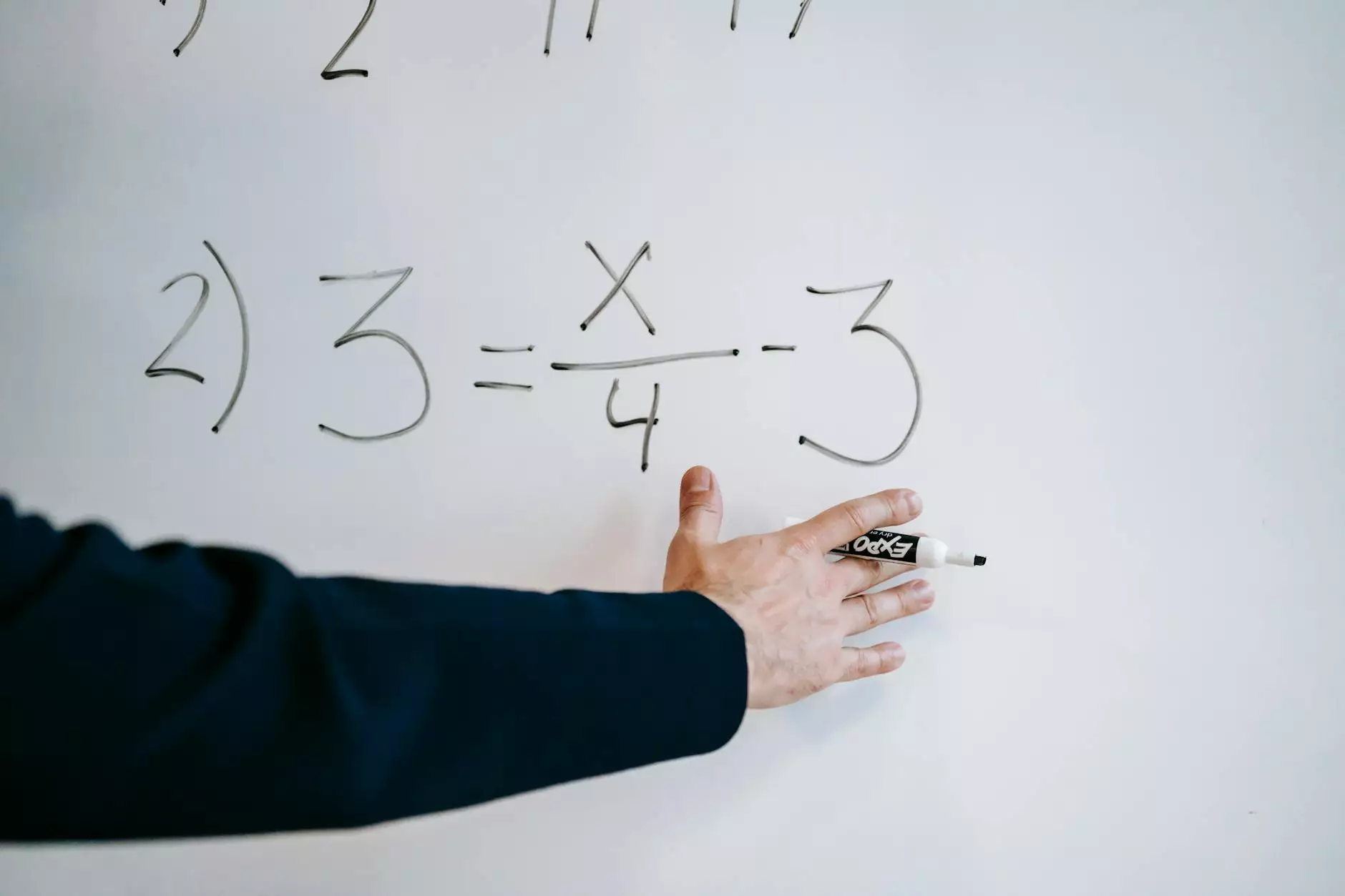
In the contemporary landscape of machine learning, the value of accurate and effective data is unparalleled. One of the pivotal aspects of preparing data for machine learning is image annotation, a critical process that enables machines to interpret and categorize visual information. This article delves into the nuances of image annotation for machine learning, exploring its necessity, methods, and how Keylabs.ai stands at the forefront of this domain.
Understanding Image Annotation
Image annotation refers to the process of labeling images to provide context to machine learning algorithms. This technique is vital for teaching machines how to understand what they see, allowing them to recognize and classify objects within images with remarkable precision. Accurate annotation sets a strong foundation for the training of algorithms, which ultimately leads to better-performing models in applications such as computer vision, automated driving, and medical imaging.
The Importance of Image Annotation in Machine Learning
Every machine learning model's effectiveness is significantly influenced by the quality of data it is trained on. Here are several reasons why image annotation is quintessential:
- Enhanced Accuracy: Precise annotations allow models to learn from correct data, reducing errors in predictions.
- Improved Feature Recognition: Annotated datasets allow algorithms to identify critical features, which enhances their capabilities in recognizing complex images.
- Dataset Diversification: Well-annotated datasets help in developing a diverse model that is robust and generalizes better across various categories.
Types of Image Annotation Techniques
Image annotation encompasses various techniques, each suitable for different applications. Understanding these techniques is essential for selecting the right approach for your machine learning project:
1. Bounding Box Annotation
This technique involves drawing rectangles around objects in images. It is widely used for tasks such as object detection, where knowing the location of an object is crucial.
2. Polygon Annotation
Polygon annotation allows for more precise marking of irregularly shaped objects. This method is highly beneficial in applications requiring detailed recognition, such as healthcare imaging.
3. Semantic Segmentation
In this technique, each pixel of the image is assigned a label, categorizing the entire image in detail, which is specifically vital for applications like autonomous driving.
4. Landmark Annotation
This technique is used predominantly in facial recognition and biometric applications, where specific features are marked and studied.
The Role of Data Annotation Tools and Platforms
To harness the potential of image annotation effectively, utilizing advanced data annotation tools and platforms is crucial. Keylabs.ai provides sophisticated, user-friendly tools designed to streamline the annotation process, making it efficient and accurate for various machine learning projects.
Features of Keylabs.ai Data Annotation Tool
- Intuitive Interface: The platform is built with user experience in mind, allowing annotators to work seamlessly.
- Multi-format Support: It supports various image formats, ensuring adaptability across different use cases.
- Collaboration Features: Team collaboration tools enable real-time updates and feedback to optimize the annotation process.
- Quality Control: Built-in quality assurance mechanisms ensure that the annotations meet high standards, reducing the need for revisions.
Steps to Effective Image Annotation
Implementing a successful image annotation project involves several key steps:
- Define Objectives: Clearly outline the goals and desired outcomes of the annotation project.
- Select Appropriate Tools: Choose the right data annotation platform, such as Keylabs.ai, that meets your specific requirements.
- Prepare the Dataset: Gather and prepare the images that need to be annotated, ensuring they are suitable for the intended application.
- Train Annotators: If working with a team, provide training to ensure that annotators understand the guidelines and standards for quality.
- Perform Annotation: Begin annotating the images according to the selected technique, keeping accuracy and consistency in mind.
- Review and Validate: Implement a review process to ensure the annotations are correct and meet the project standards.
- Integrate with ML Models: After validation, the annotated images can be used to train machine learning models.
Challenges in Image Annotation
While essential, image annotation presents its own set of challenges.
- Scalability: As datasets grow, maintaining high-quality annotations becomes challenging.
- Labor-Intensive: Manual annotation can be time-consuming and costly, requiring a considerable workforce.
- Subjectivity: Annotation can be subjective, leading to inconsistencies if not properly managed.
- Complexity of Multilabel Cases: Some images may contain multiple objects or concepts that need careful annotation.
Future Trends in Image Annotation for Machine Learning
The evolution of image annotation is intertwined with advancements in technology. Here are some noteworthy trends shaping the future of this field:
1. Automation and AI-assisted Annotation
With the rise of artificial intelligence, automated annotation tools are becoming increasingly sophisticated, significantly reducing the time and effort needed for large datasets.
2. Use of Synthetic Data
Generating synthetic data for training can enhance models without requiring expansive real-world datasets, and it necessitates corresponding synthetic annotations.
3. Crowd-sourced Annotation
Leveraging crowd-sourcing platforms allows for a diverse range of input, enhancing the richness and depth of annotated data.
4. Real-time Annotation Systems
Future platforms may offer real-time annotation capabilities, making it easier for teams to collaborate and iterate quickly on models.
Conclusion
Image annotation for machine learning is an indispensable component in developing effective and advanced machine learning algorithms. By providing detailed, accurate, and varied data, image annotation empowers machines to evolve and adapt in ways that were previously unimaginable. As technology continues to advance, so too will the methods and tools used for image annotation, paving the way for innovations that will drive the future of machine learning.
At Keylabs.ai, we are committed to leading the charge in image annotation by providing superior tools and platforms that enhance the annotation process. Our goal is to help companies unlock the full potential of their datasets, ensuring that they remain competitive in the ever-evolving landscape of artificial intelligence and machine learning.