The Importance of Bounding Box in Data Annotation
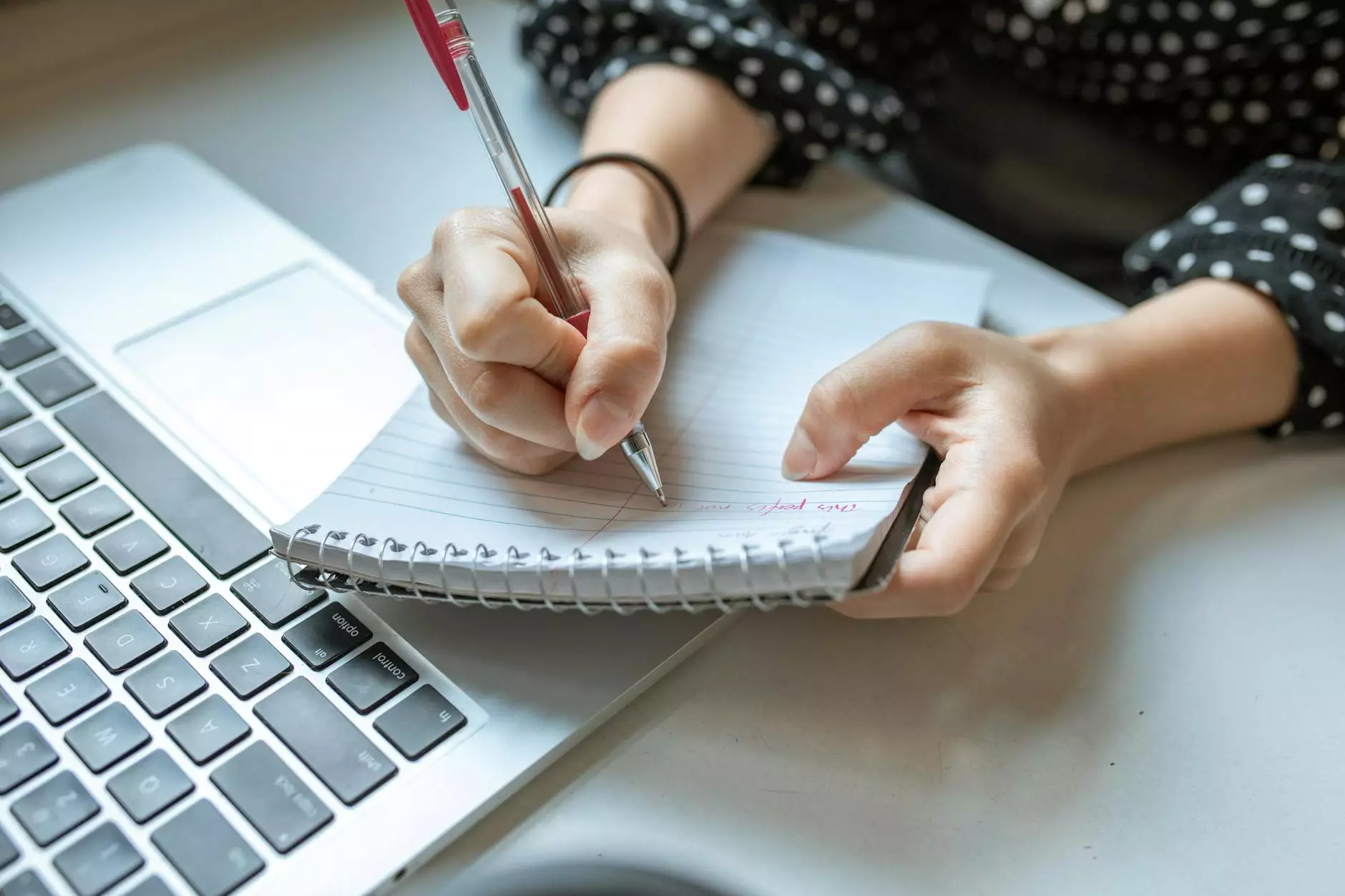
In the rapidly evolving landscape of artificial intelligence and machine learning, the significance of accurate data annotation cannot be overstated. One of the most crucial techniques in this realm is the concept of the bounding box. As businesses strive to leverage AI capabilities, understanding the role of bounding boxes in data annotation tools and platforms becomes essential. In this comprehensive article, we will delve into what a bounding box is, its importance in various applications, particularly in computer vision, and how platforms like KeyLabs AI can help businesses implement this technique effectively.
What is a Bounding Box?
A bounding box is defined as a rectangle that is drawn around an object in an image or video frame. This rectangle is used to identify and locate the object within the given space. It acts as a simple yet effective way to annotate images for tasks such as object detection, image classification, and segmentation. The bounding box typically consists of four coordinates: the horizontal and vertical positioning of the top-left corner, and the width and height that determine the rectangle’s size.
Why Are Bounding Boxes Important?
Bounding boxes are pivotal in data annotation because they form the foundational building blocks of machine learning models designed for visual tasks. Here are a few reasons highlighting their importance:
- Efficiency in Object Detection: Bounding boxes allow for efficient training of object detection models, enabling computers to recognize and classify various objects accurately.
- Simplicity in Annotation: They provide a straightforward way to mark the location of objects, making the annotation process faster and easier for human labelers.
- Foundation for Further Analysis: Once an image or video is annotated with bounding boxes, it sets the stage for additional analysis such as facial recognition, vehicle detection, and much more.
- Standardization: Using bounding boxes creates a standardized method for annotating image data, which is essential for training robust AI systems.
Applications of Bounding Boxes in Various Industries
The application of bounding boxes spans across multiple industries, each benefiting from the precision and reliability they provide in data annotation. Below are several fields where bounding boxes are notably impactful:
1. Autonomous Vehicles
In the automotive sector, especially with the rise of autonomous driving technologies, bounding boxes are critical. They help in recognizing pedestrians, cars, traffic signs, and obstacles on the road. Training AI models using annotated images with bounding boxes enhances the safety and efficiency of self-driving vehicles.
2. Healthcare
In medical imaging, bounding boxes can be used to identify and segment specific areas of interest, such as tumors in MRI or CT scans. This precise localization aids radiologists in diagnosing conditions more accurately and at earlier stages.
3. Retail and Inventory Management
Bounding boxes are also applied in retail environments, particularly in inventory management solutions. They assist in object detection algorithms that can monitor stock levels, helping retailers maintain optimal inventory and reduce losses.
4. Security and Surveillance
In security applications, bounding boxes enable the detection of individuals or objects in surveillance footage. This technology supports alert systems in real-time, greatly enhancing security measures in public and private spaces.
5. Sports Analytics
Sports analytics companies utilize bounding boxes to track player movements and ball trajectories in game footage. This data helps teams improve strategies through detailed analysis, enhancing performance on the field.
The Process of Using Bounding Boxes in Data Annotation
The process of implementing bounding boxes in data annotation involves several key steps:
- Data Collection: Gather a diverse set of images or video data relevant to the task at hand.
- Annotation Guidelines: Define clear guidelines on how to create bounding boxes for consistency among annotators.
- Annotation Tools: Employ data annotation tools that support bounding box creations, such as the platforms provided by KeyLabs AI.
- Quality Assurance: Regularly check the annotated data to ensure accuracy and consistency, correcting any discrepancies.
- Integration into ML Models: Utilize the annotated data to train machine learning models effectively.
Challenges in Bounding Box Annotation
While bounding box annotation is essential, there are challenges associated with it:
- Complex Object Shapes: Bounding boxes may struggle to accurately encapsulate irregularly shaped objects, leading to potential misclassifications.
- Occlusions: When objects overlap, it can be challenging to create a bounding box that accurately represents the visible part of the object.
- Consistency Across Annotators: Different annotators may have varying perspectives on where to draw bounding boxes, leading to inconsistencies in the annotated dataset.
Best Practices for Bounding Box Annotation
To maximize the effectiveness of bounding box annotations, businesses should consider these best practices:
- Training Annotators: Provide comprehensive training for individuals tasked with data annotation to align understanding of guidelines and expectations.
- Utilizing Robust Tools: Invest in high-quality data annotation tools that facilitate the efficient creation and management of bounding boxes.
- Regular Review and Feedback: Conduct periodic reviews of annotations and offer constructive feedback to improve accuracy over time.
- Maintaining Diversity in Data: Ensure the dataset includes varied examples to build models that are resilient to different scenarios.
The Role of KeyLabs AI in Data Annotation
KeyLabs AI stands at the forefront of providing advanced data annotation tools and platforms that facilitate the efficient creation of bounding boxes. Their platform offers a user-friendly interface, comprehensive annotation tools, and integration capabilities that ensure high-quality data preparation for AI training. Here’s how KeyLabs AI can empower businesses:
Streamlined Annotation Process
The tools provided by KeyLabs AI allow businesses to streamline their annotation process, reducing the time and effort involved while increasing the overall quality of the annotated data.
Collaborative Environment
KeyLabs AI fosters a collaborative environment where teams can work together on data annotation projects, ensuring consistency and quality in the output.
Integration with ML Workflows
The platform seamlessly integrates with machine learning workflows, allowing businesses to move from data collection to model training and deployment without unnecessary bottlenecks.
Expert Support and Resources
KeyLabs AI provides expert support and resources, ensuring businesses can make the most of their annotation tools and achieve optimal results in their AI initiatives.
Conclusion
In conclusion, the concept of the bounding box is integral to the success of various AI applications through effective data annotation. From autonomous vehicles to healthcare analytics, the advantages of utilizing bounding boxes cannot be understated. As organizations aim to harness the power of AI, leveraging robust data annotation platforms like KeyLabs AI will provide the necessary foundation to build reliable machine learning models. By following best practices and overcoming common challenges, businesses can fully exploit the capabilities of bounding boxes, enhancing their operations and driving innovation in their respective fields.